Table Of Content
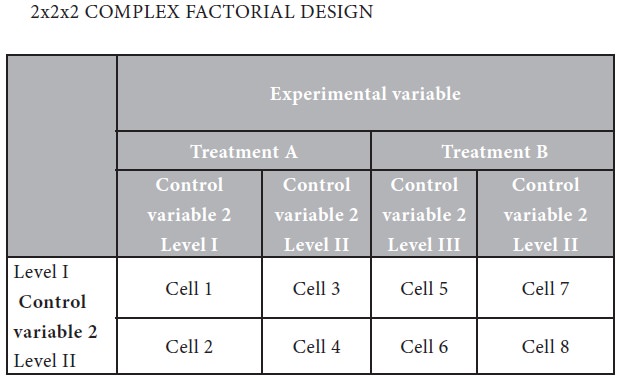
You also notice that age does not play a role; both 20 and 40 year olds suffer the same percentage of seizures for a given amount of CureAll. From this information, you can conclude that the chance of a patient suffering a seizure is minimized at lower dosages of the drug (5 mg). The second graph illustrates that with increased drug dosage there is an increased percentage of seizures, while the first graph illustrates that with increased age there is no change in the percentage of seizures.
4. Complex Correlational Designs¶
This paper is intended to alert the investigator to such challenges as this may inform decisions about whether to use a factorial design, and how to do so. This paper will use smoking treatment research to illustrate its points, but its content is broadly relevant to the development and evaluation of other types of clinical interventions. Also, it will focus primarily on research design and design implementation rather than on statistical analysis (for relevent discussion of statistical analysis see Box, Hunter, & Hunter, 2005; Keppel, 1991).
2: Design of experiments via factorial designs
Aquaculture rearing systems induce no legacy effects in Atlantic cod larvae or their rearing water bacterial communities ... - Nature.com
Aquaculture rearing systems induce no legacy effects in Atlantic cod larvae or their rearing water bacterial communities ....
Posted: Thu, 17 Nov 2022 08:00:00 GMT [source]
Only first order terms were included in the analysis to create a linear model. Pareto charts for both wt% MeOH in biodiesel and number of theoretical stages are shown below. In the Graphs menu shown above, the three effects plots for "Normal", "Half Normal", and "Pareto" were selected.
Table 1.
The best they can do is show patterns of relationships that are consistent with some causal interpretations and inconsistent with others. The example in Figure 5.15 shows a case in which it is probably a bit more straightforward to interpret both the main effects and the interaction. You can look at the red bars first and see that the red bar for no-shoes is slightly smaller than the red bar for shoes.
Although the full factorial provides better resolution and is a more complete analysis, the 1/2 fraction requires half the number of runs as the full factorial design. In lack of time or to get a general idea of the relationships, the 1/2 fraction design is a good choice. Additionally, the number of center points per block, number of replicates for corner points, and number of blocks can be chosen in this menu. For a 2 level design, click the "2-level factorial (default generators)" radio button. Other designs such as Plackett-Burman or a General full factorial design can be chosen.
Including numerous factors might also increase the occurrence of interactions, which might affect the magnitude of a main effect (despite the lack of correlation between main and interaction effects with effect coding). Finally, including numerous ICs in an experiment could cause staff or counselors to spontaneously adjust their delivery of an intervention component because of their awareness of the total intensity of treatment provided to a participant. Counselors could either reduce the intensity of an intervention component when it is one of many that a participant receives, or they could increase the intensity of an intervention component if the participant is receiving little other treatment. In short, maintaining treatment delivery fidelity may take more care, training and supervision in a factorial experiment than in an RCT. Thus, two different active treatments might be contrasted with one another in a two-group design, such as a comparison of two different counseling approaches (e.g., skill training vs. supportive counseling), each paired with the same medication.
Similarly, the two contrast vectors for B depend only on the level of factor B, namely the second component of "cell", so they belong to the main effect of B. For example, a shrimp aquaculture experiment[9] might have factors temperature at 25°C and 35°C, density at 80 or 160 shrimp/40 liters, and salinity at 10%, 25% and 40%. In many cases, though, the factor levels are simply categories, and the coding of levels is somewhat arbitrary. For example, the levels of an 6-level factor might simply be denoted 1, 2, ..., 6. From this one can see that there is an interaction effect since the lines cross.
This main total effect value for each variable or variable combination will be some value that signifies the relationship between the output and the variable. For instance, if your value is positive, then there is a positive relationship between the variable and the output (i.e. as you increase the variable, the output increases as well). So while the largest main total effect value in one set of experiments may have a value of 128, another experiment may have its largest main total effect value be 43. By the traditional experimentation, each experiment would have to be isolated separately to fully find the effect on B. Note that only four experiments were required in factorial designs to solve for the eight values in A and B. In the previous section, we looked at a qualitative approach to determining the effects of different factors using factorial design.
For instance, in the design depicted in Table 1, the effect of Extended Medication would be reflected by the average effect of all Extended Medication conditions (1–16) versus the average effect of all Standard Medication conditions (17–32). With effect coding, when the experimental conditions have equal (or nearly equal) numbers of participants, the main effect of a factor does not reflect the effects of interaction effects that may be present in the data. In general, if the major goal of a study is to contrast directly one “treatment” with another treatment (e.g., a control treatment), then an RCT is usually the best choice. Note that here “treatment” is used to connote a set of intervention components (ICs); e.g., a particular type, dose, and duration of medication, type of counseling that is delivered for a particular number of sessions of a particular duration, and so on. The statistical analyses would reveal whether the experimental treatment “package” differs in effects from the usual care treatment.
Typically, if the same experimentation will occur for 3 lab periods, 2 replicates will be added. Replicates are repeats of each trial that help determine the reproducibility of the design, thus increasing the number of trials and accuracy of the DOE. To add replicates, click the "Replicate design" radio button in the "Modify Design" menu. The examples discussed in this section only scratch the surface of how researchers use complex correlational research to explore possible causal relationships among variables. It is important to keep in mind, however, that purely correlational approaches cannot unambiguously establish that one variable causes another.
Like Pareto plots, Half Normal plots show which factors have significant effects on the responses. The factors that have significant effects are shown in red and the ones without significant effects are shown in black. The further a factor is from the blue line, the more significant effect it has on the corresponding response. For wt% methanol in biodiesel, RPM is further from the blue line than pressure, which indicates that RPM has a more significant effect on wt% methanol in biodiesel than pressure does. Factors A - D can be renamed to represent the actual factors of the system.
The between-subjects design is conceptually simpler, avoids carryover effects, and minimizes the time and effort of each participant. The within-subjects design is more efficient for the researcher and help to control extraneous variables. In many factorial designs, one of the independent variables is a non-manipulated independent variable.
Hence, the case and control are matched on calendar time and length of follow‐up. When this study design is implemented, it is possible for the control that was selected early in the study to develop the disease and become a case in the latter part of the study. In other words, sunlight and watering frequency do not affect plant growth independently. Rather, there is an interaction effect between the two independent variables. A factorial experimental design is an experimental design that is used to study two or more factors, each with multiple discrete possible values or “levels”.
Exploring hydrothermal liquefaction (HTL) of digested sewage sludge (DSS) at 5.3 L and 0.025 L bench scale using ... - Nature.com
Exploring hydrothermal liquefaction (HTL) of digested sewage sludge (DSS) at 5.3 L and 0.025 L bench scale using ....
Posted: Wed, 01 Nov 2023 07:00:00 GMT [source]
Indeed, even without the use of special assessments, investigators might correlate the number of ICs a person receives (regardless of type) to outcomes. Factorial designs require the experimenter to manipulate at least two independent variables. Imagine you are trying to figure out which of two light switches turns on a light. The first independent variable is light switch #1, and it has two levels, up or down. The second independent variable is light switch #2, and it also has two levels, up or down.
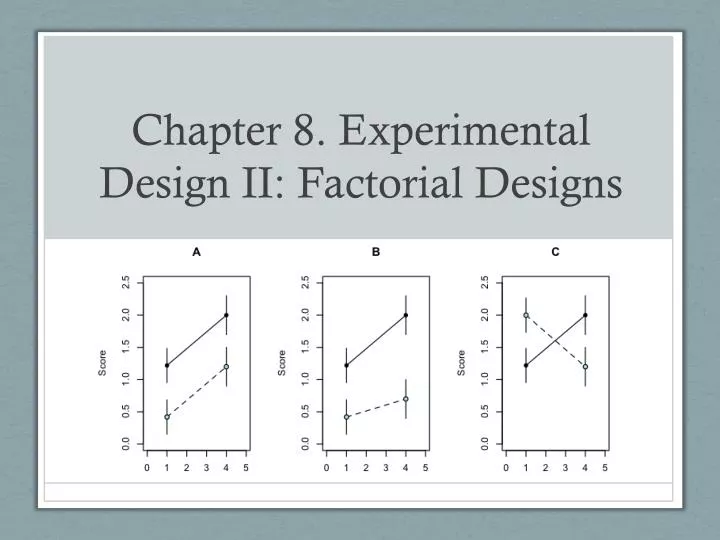
For example, does the effect of time since last meal depend on the levels of the tired variable? Look first at the effect of time since last meal only for the red bars in the “not tired” condition. The red bar in the 1 hour condition is 1 unit smaller than the red bar in the 5 hour condition. Next, look at the effect of time since last meal only for the green bars in the “tired” condition. The green bar in the 1 hour condition is 3 units smaller than the green bar in the 5 hour condition. Again, the size of the effect of time since last meal depends on the levels of the tired variable.No matter which way you look at the interaction, we get the same numbers for the size of the interaction effect, which is 2 units (i.e., the difference between 3 and 1).
If they were high in private body consciousness, then those in the messy room made harsher judgments. If they were low in private body consciousness, then whether the room was clean or messy did not matter. Finally, it is important to note that if investigators include multiple, discrete IC’s in a factorial experiment the effects of the individual ICs may be limited to the extent that the various ICs exert their effects via similar or redundant pathways (Baker et al., 2016). Thus, to the extent that two ICs affect coping execution or withdrawal severity, their co-occurrence in the experiment could reduce estimates of their main effects via negative interaction.
No comments:
Post a Comment